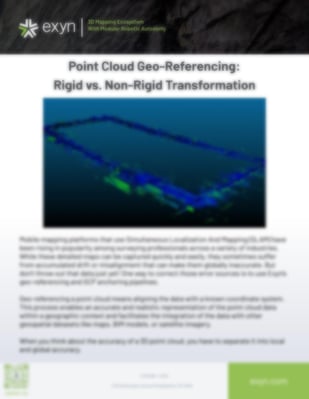
Mobile mapping platforms that use Simultaneous Localization And Mapping (SLAM) have been rising in popularity among surveying professionals across a variety of industries. While these detailed maps can be captured quickly and easily, they sometimes suffer from accumulated drift or misalignment that can make them globally inaccurate. But don't throw out that data just yet!
In this whitepaper we will cover:
-
Various methods for post-processing point clouds
-
The differences between rigid & non-rigid transformations
-
How surveyors can use this information to better plan for data capture in the field
Unpack The Power of SLAM
Download your copy of
Point Cloud Geo-Referencing: Rigid vs. Non-Rigid Transformation